Dedicated EndpointsBuild and run LLMs/LMMs on autopilot
Autopilot LLM endpoints for production
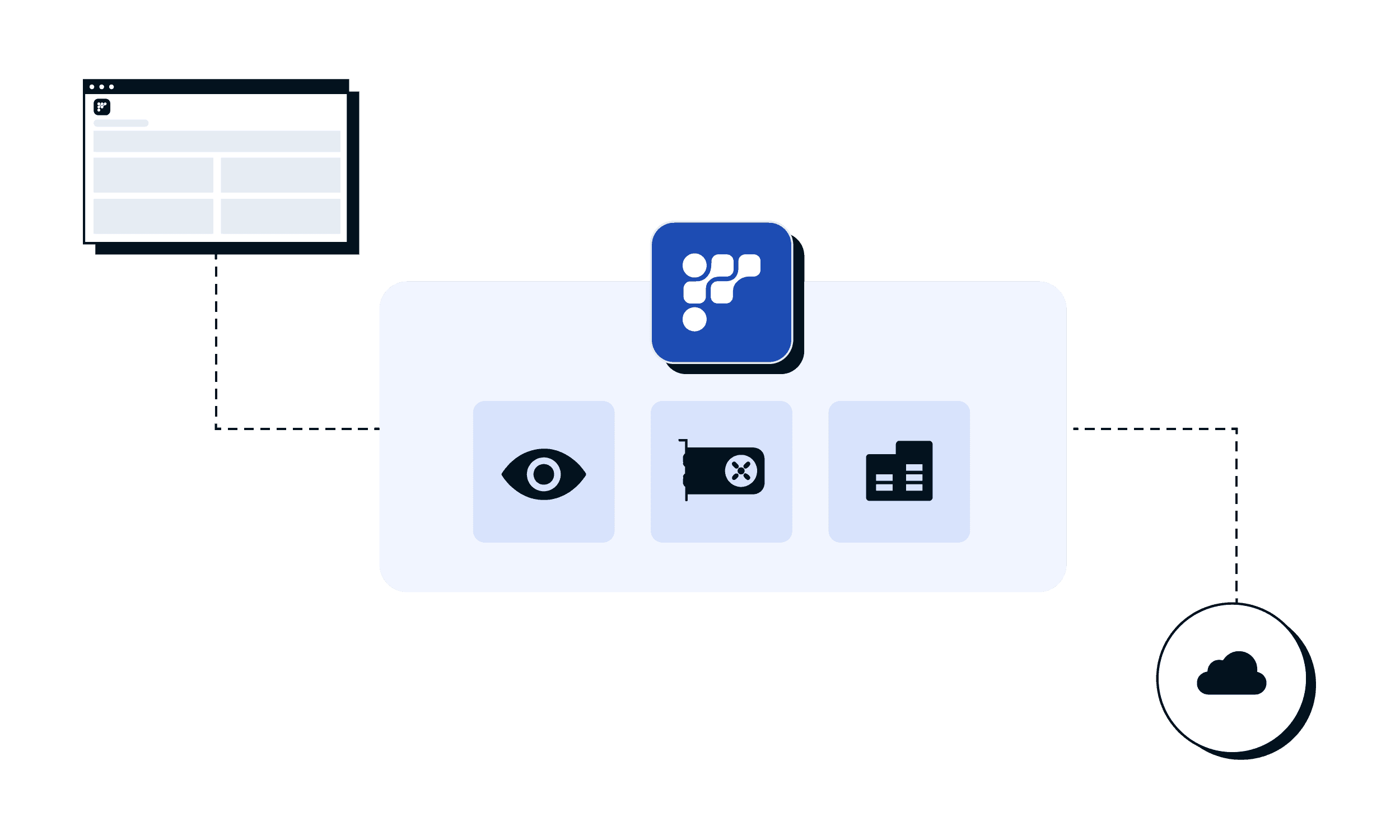
“Working with FriendliAI, we created a
convenient and dependable service
without the need for self-management”
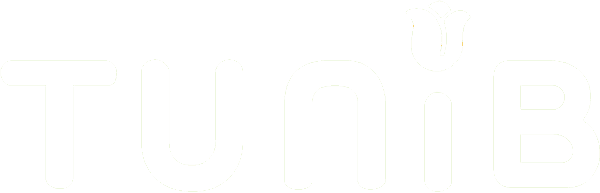
Now you can find Friendli Dedicated Endpoints on AWS marketplace and making LLM building and serving seamless and efficient.
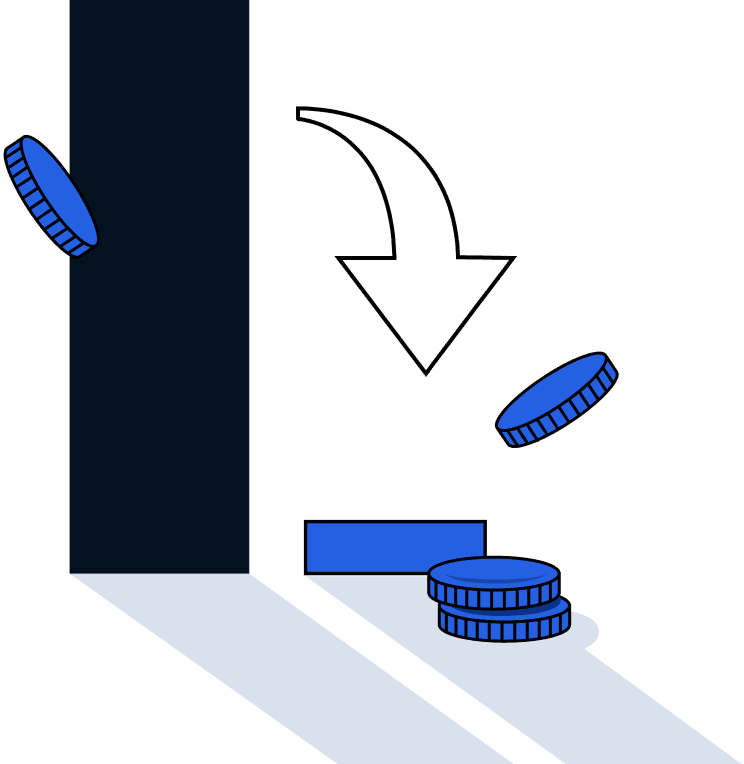
Superior cost-efficiency
and performance
Having a performant LLM serving solution is the first step to operate your AI application in the cloud.
Custom model support
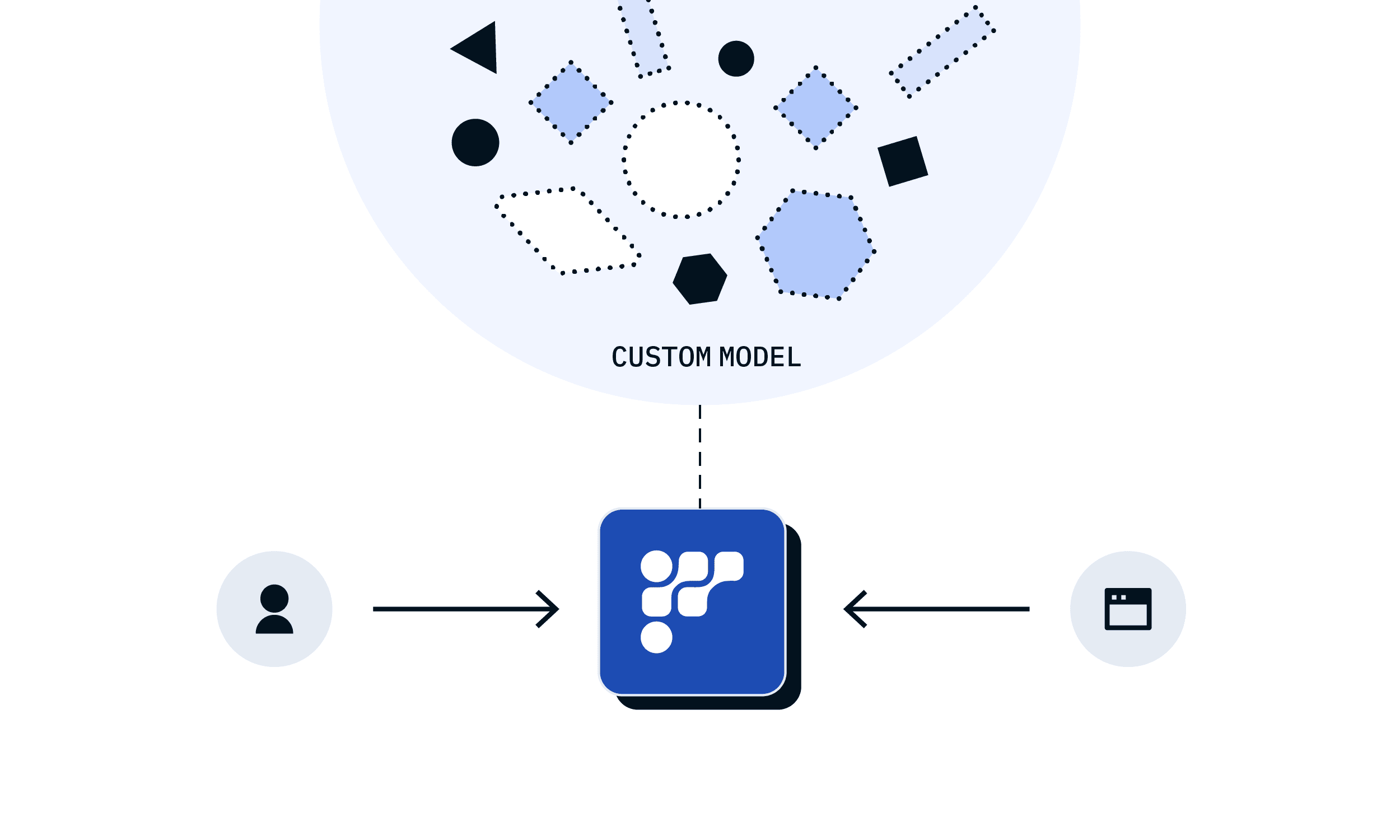
We offer comprehensive support for both open-source and custom LLMs, allowing organizations to deploy models tailored to their unique requirements and domain-specific challenges.With the flexibility to integrate proprietary datasets, businesses can unlock new opportunities for innovation and differentiation in their AI-driven applications.Create a new endpoint with your private Hugging Face Model Hub repository or upload your model directly to Dedicated Endpoints.
Dedicated GPU Resource Management
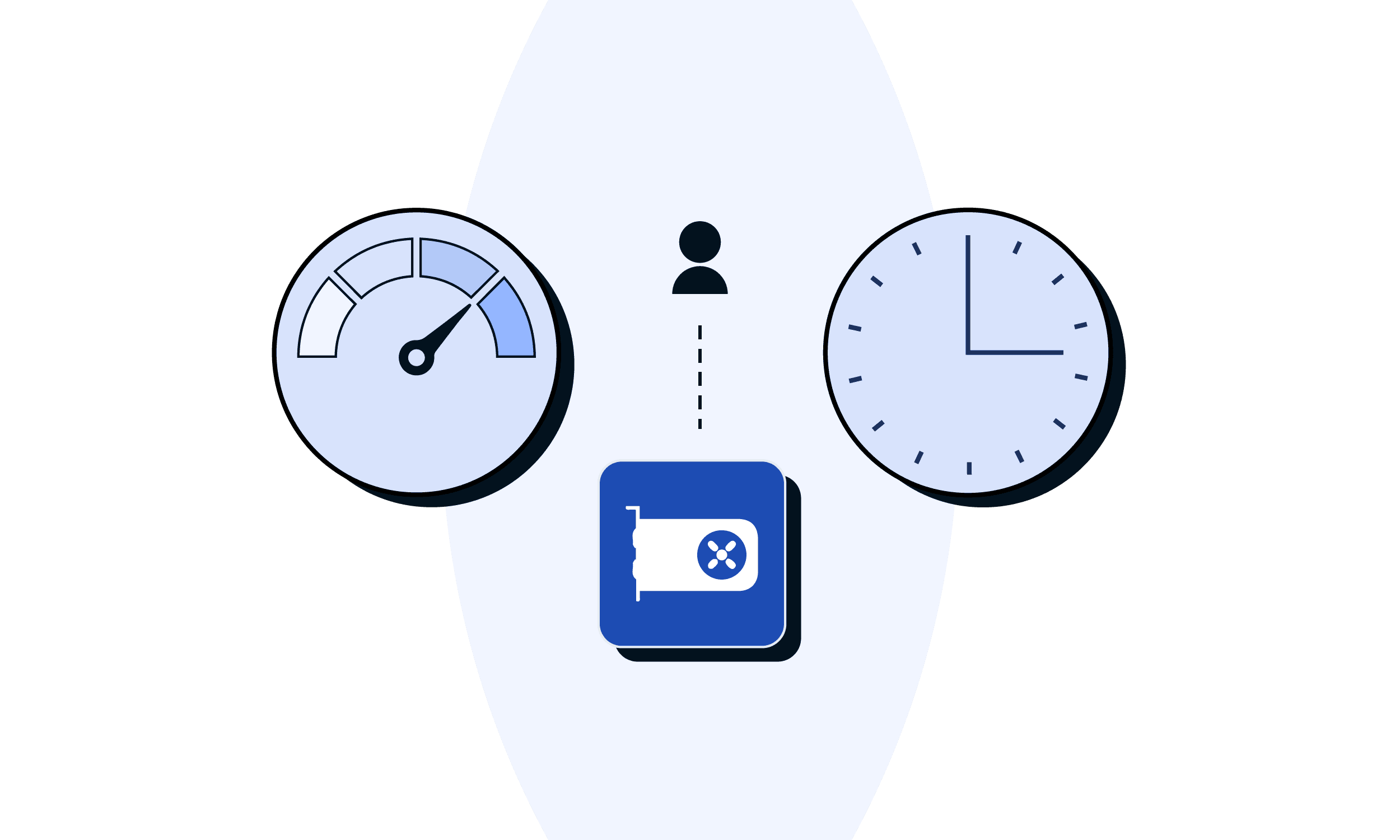
FriendliAI Dedicated Endpoints provides dedicated GPU instances ensuring consistent access to computing resources without contention or performance fluctuations.By eliminating resource sharing, organizations can rely on predictable performance levels for their LLM inference tasks, enhancing productivity and reliability.
Auto-Scale your resources on cloud
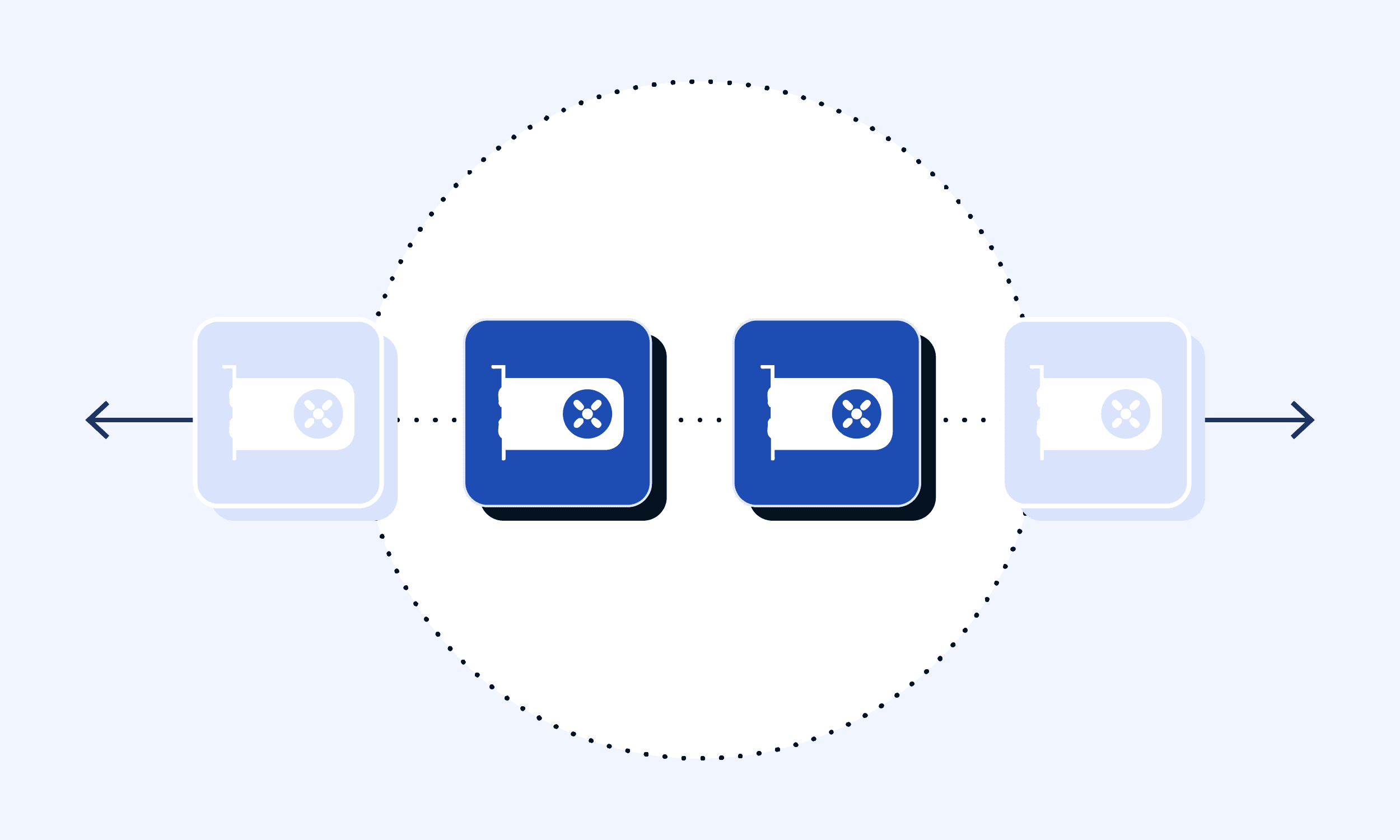
When deploying generative AI on cloud, it is important to scale as your business grows.Friendli Dedicated Endpoints employs intelligent auto-scaling mechanisms that dynamically adjust computing resources based on real-time demand and workload patterns.
Basic
Sign upGet $10 in free credits upon sign up
Build and run LLMs/LMMs on autopilot
Billed monthly
Friendli on A100 80GB
$3.8 per hour
Friendli on H100 80GB
$7.6 per hour
Enterprise
Contact SalesCustom pricing
Dedicated support
Other ways to run generative AI models with Friendli
TECH BLOG
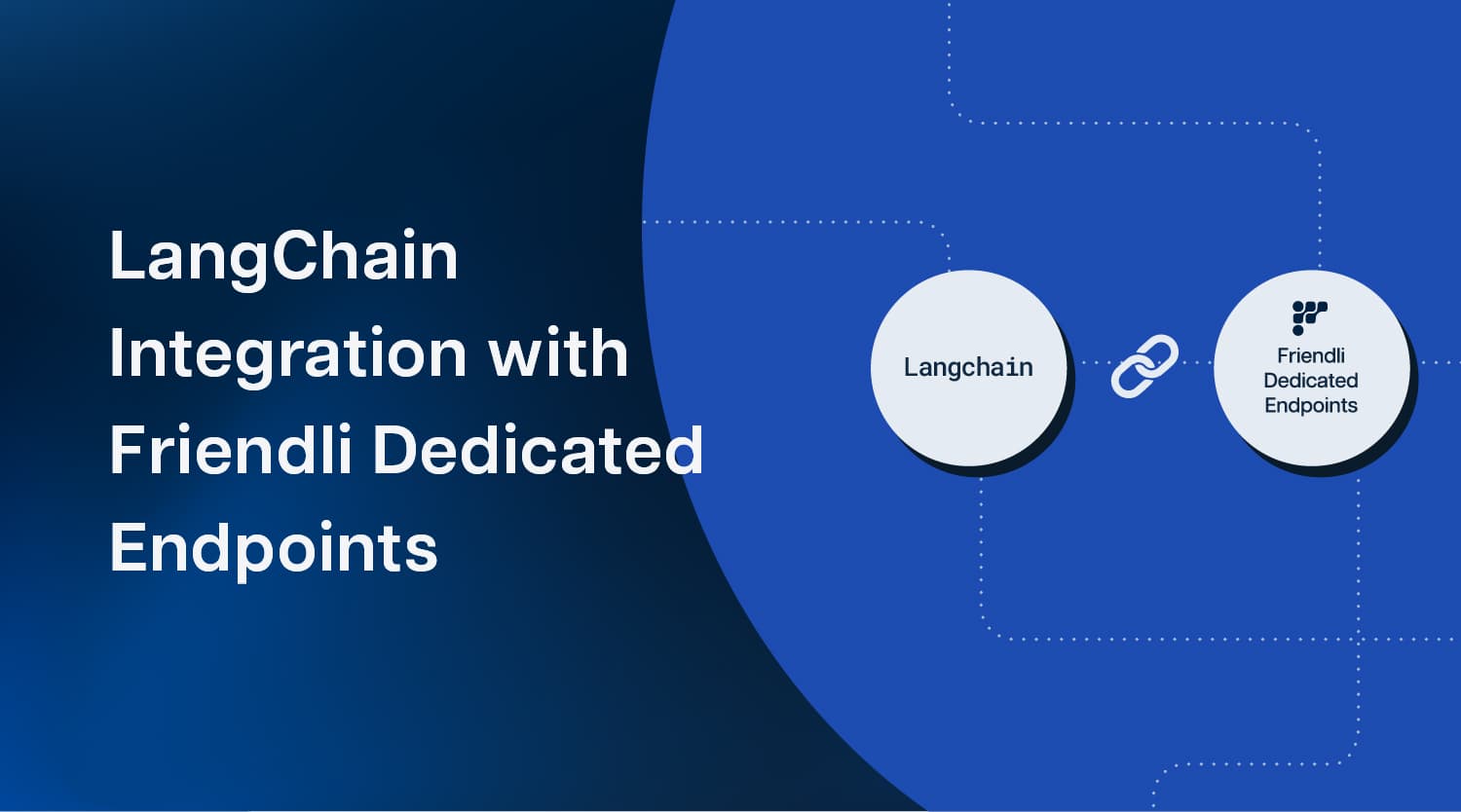
LangChain Integration with Friendli Dedicated Endpoints
In this article, we will demonstrate how to use Friendli Dedicated Endpoints with LangChain. Friendli Dedicated Endpoints is our SaaS service for deploying generative AI models that run Friendli Engine, our flagship LLM serving engine, on various cloud platforms. LangChain is a popular framework for building language model applications. It offers developers a convenient way of combining multiple components into a language model application. Using Friendli Dedicated Endpoints with LangChain allows developers to not only write language model applications easily, but also leverages the capabilities of Friendli Engine, our flagship LLM serving engine, to enhance the performance and cost-efficiency of serving the LLM model. ### Building a Friendli LLM interface for LangChain LangChain provides various LLM model interfaces and also allows defining a custom interface with ease by inheriting LangChain’s base LLM model. First, to get started, you'll need a running Friendli Engine deployment and an API key. Please refer to our docs for running a deployment on Friendli Dedicated Endpoints. Then, Friendli Engine provides a Python SDK for running language completion tasks, so we’ll use its completion API to implement our custom interface. Here is our Friendli Engine LLM interface for LangChain: ```python from langchain.llms.base import LLM from langchain.schema import LLMResult from friendli import Completion, V1CompletionOptions class FriendliEndpoint(LLM): """Friendli LLM interface api_key: Friendli Dedicated Endpoints API Key endpoint: Friendli Dedicated Endpoints deployment endpoint option: Text completion options. Please check out https://docs.friendli.ai/openapi/create-completions for full options """ api_key: str | None = None endpoint: str = "" options: dict = dict( max_tokens=200, top_p=0.8, temperature=0.5, no_repeat_ngram=3, ) @property def _llm_type(self) -> str: """Return type of llm.""" return "friendli" def _call( self, prompt: str, stop: list[str] | None = None, run_manager: CallbackManagerForLLMRun | None = None, kwargs: Any, ) -> str: """LLM inference method.""" options = V1CompletionOptions( prompt=prompt, stop=stop, self.options, ) # Define an API endpoint instance api = Completion(endpoint=self.endpoint, deployment_security_level="public") # Requests text generation to Friendli Dedicated Endpoints deployment completion = api.create(options=options, stream=False) return completion.choices[0].text # Returns generated text ``` Now we can simply create an instance and use it like any other LLMs in the LangChain framework: ```python friendli_llm = FriendliEndpoint( api_key="FRIENDLI_API_KEY", endpoint="https://friendli-deployment-endpoint", ) friendli_llm.predict("Python is a popular") # >> "general-purpose programming language that supports..." ``` ### Streaming Friendli Engine also supports streaming a response, so that instead of waiting for the full response, you can receive intermediate results during generation. The LangChain framework also supports the streaming interface as \_stream and \_astream method, so we’ll also implement them using Friendli Engine's stream option. ```python from langchain.schema.output import GenerationChunk class FriendliDeployement(LLM): ... def _stream( self, prompt: str, stop: list[str] | None = None, run_manager: CallbackManagerForLLMRun | None = None, kwargs: Any, ) -> Iterator[GenerationChunk]: options = V1CompletionOptions( prompt=prompt, stop=stop, self.options, ) """LLM inference method with streaming option.""" api = Completion(endpoint=self.endpoint, deployment_security_level="public") stream = api.create(options=options, stream=True) # Requests generation with streaming option for line in stream: # Receives and returns generated tokens in streaming fashion chunk = GenerationChunk(text=json.dumps(line.model_dump())) yield chunk if run_manager: # If the callback manager is given, invokes its token handler run_manager.on_llm_new_token(line.text, chunk=chunk) ``` With the streaming interface, you can display the response to the user as it’s being generated in real-time: ```python from friendli.schema.api.v1.completion import V1CompletionLine async for resp in friendli_llm.astream("Tell me a story"): line = V1CompletionLine.model_validate_json(resp) print(line, end="") # Asynchronously prints generated tokens ``` In summary, we’ve implemented a custom Friendli Engine LLM interface for LangChain and looked at how it can be used with basic examples. In our next blog post, we will see how to build more complex LLM applications using the Friendli Engine and LangChain. Get started today with Friendli Engine!
Read more